Putting a Halt to the Insanity Surrounding Data Access for Healthcare AI Development & Deployment
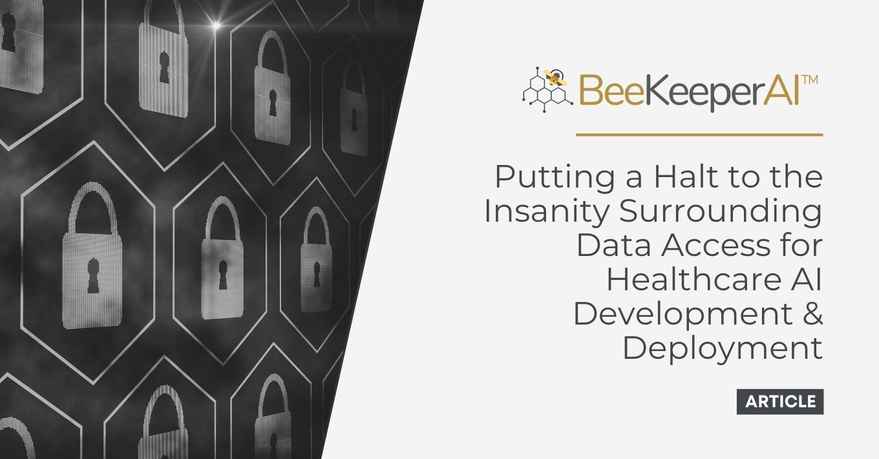
By Mary Beth Chalk, Co-founder and Chief Commercial Officer, BeeKeeperAI
At BeeKeeperAI, we are developing innovative tools for healthcare artificial intelligence lifecycle management that will ultimately improve patient outcomes and reduce treatment costs. As experts in the field of healthcare AI, we understand the difficulties of securing real-world data in quantities needed to produce models that perform consistently once they have been deployed. It is a time-consuming and costly process for algorithm developers.
Given the data access challenges, it is not surprising that many AI algorithms have performance problems. We have all seen the articles that highlight inherent biases in data that reduce the accuracy of healthcare AI models, lack of generalizability, as well as issues with algorithm drift when the models are exposed to new data.
Last year, a VentureBeat article reported on problems with machine learning algorithms developed to detect and diagnose COVID-19 cases from imaging data. The article cited a study originally published in Nature Machine Intelligence in which a team of healthcare professionals and AI experts analyzed machine learning models described in 62 papers. They concluded that none of the models were “likely candidates for clinical translation for the diagnosis/prognosis of COVID-19.”
We were not at all surprised by those results. Here’s our take on the findings and why they are to be expected.
We were not surprised to learn that roughly half of the COVID and pneumonia detection models received no external validation. Before algorithms can be used in clinical settings, developers must show that they perform in an ethnically, clinically, and geographically agnostic manner in varied healthcare settings. To get that kind of generalizability, models must be trained and validated on all possible variables they are likely to experience when deployed. Getting that much data under normal conditions is hard enough. During the pandemic, it would have been almost impossible.
We were not surprised by the creative measures (e.g., “Frankenstein” datasets) that developers used to overcome their data access issues. It can take 18-24 months to construct sufficiently diverse data sets (both for training and validation) to develop generalizable healthcare AI. And it is not cheap! Increasing risks of cyberattacks on the healthcare have amplified the costs and complexity of accessing needed data. Estimates suggest that it could cost as much as $2.5M or more per model. Is it any wonder that algorithm developers are considering short-cuts to get the data they need?
We were not surprised that algorithm developers were unwilling to expose their model to third parties to validate the performance. Existing methods for supplying algorithms with training data have ample avenues where intellectual property could be exposed. Scientists spend months and years designing and refining their algorithms. Given the capital and human labor required to build these models, companies are understandably concerned about risks to IP especially if it is a core component of their business.
The market for healthcare AI is expected to surpass $67 B by 2027. To achieve its full potential, algorithm developers and data stewards need innovative solutions that enable them to interact with each other securely and safely. Traditional federated learning methods are not sufficient. We need an entirely new paradigm.
That is why we developed BeeKeeperAITM a zero trust, confidential computing platform that enables secure collaboration between algorithm developers and those entrusted to protect personal health information, the data stewards. We’ll be talking more about our technology and vision in future posts but for now, here are some of the highlights of our offering:
- Data stewards’ data never leaves the HIPAA-protected cloud environment.
- Data stewards’ data is never shared nor exposed for attack.
- Algorithm developers never have to expose their code base or model weights to third parties.
With our technology, algorithm developers get enough prospective and retrospective data to properly train their algorithms. And data stewards have a way to share information without worrying about unintentionally compromising patient privacy. To learn more, click here to get in touch with us.
0 comments
Leave a comment
Please log in or register to post a comment