Securing Healthcare AI with Confidential Computing
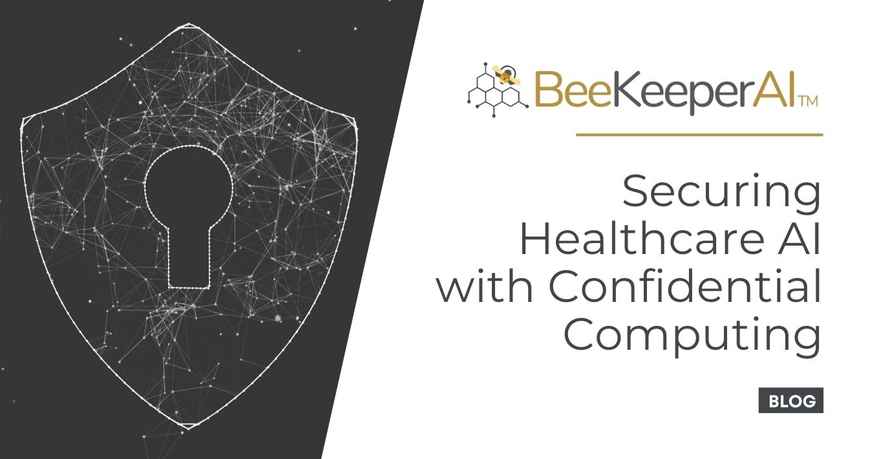
The following guest post is re-posted with permission from Richard Searle, Customer Solutions Director, Fortanix.
Contextual Focus on Healthcare
The events of 2020 have placed healthcare and medical research at the forefront of public discourse and topical interest. The quest for an effective vaccine to counter the severe acute respiratory syndrome coronavirus 2 (SARS-Cov-2) virus and its manifestation in the disease COVID-19 has introduced sampling, statistical analysis, and the reproduction coefficient (R) as themes within everyday conversation.
In the search for new medicines and clinical therapies to treat and prevent COVID-19, vast quantities of data have been gathered and processed. This accumulation of data has provided information about diverse factors such as the molecular structure of the SARS-Cov-2 virus and the demographic composition of patient groups at particular risk from COVID-19. Data has been collected, aggregated, and disaggregated at regional, national, and international levels of analysis. Within this growing repository of data, protected healthcare information (PHI) is intertwined with other sensitive information, for example social interactions disclosed by track-and-trace applications that are subject to stringent data privacy laws. Public awareness of the type and scale of the data being generated and applied within healthcare has raised legitimate questions about the security of the gathered data and the scope of the data privacy regulations that govern its use.
Demonstrated Benefits of AI in Healthcare and the Life Sciences
The benefits to be realized through the application of artificial intelligence (AI) in healthcare and the life sciences can be contextualized through the breakthrough in the prediction of protein structures made by the DeepMind AlphaFold system. The latest version of AlphaFold achieves a median score of 92.4 for the Global Distance Test (GDT) used to evaluate the accuracy of predicted protein folding versus the form of the actual protein [1].
Using experimental data [2], the researchers at DeepMind were able to confirm the accuracy of the AlphaFold predictions obtained for the structure of the SARS-Cov-2 trimeric spike protein. This finding enabled early prediction of additional SARS-Cov-2 proteins [3], providing valuable insights for laboratory research, without the initial requirement for iterative experimentation. The reduction in the amount of time and resources required to achieve meaningful research that is made possible by the AlphaFold system, coupled with the potential for genuine scientific advancement through new insights into understudied or unknown protein structures, clearly demonstrates how AI can bring transformational benefits to the field of healthcare and life sciences.
Within clinical settings, AI can support medical professionals by processing volumes of data and determining diagnoses that are beyond the capability of knowledge of the clinician. Topol [4] cites the case of an eight-day-old baby boy admitted to hospital presenting with continual seizures, known as status epilepticus – a life threatening condition. A series of machine systems analyzed 125 gigabytes of data to sequence the boy’s whole genome. Within 20 seconds, a natural language processing (NLP) AI algorithm defined 88 phenotype features from the child’s medical record (compared to only 20 features identified by the attendant medical team). Using this data, downstream algorithms sifted five million genetic variations, which, combined with the patient’s phenotypic data, enabled these AI systems to identify the candidate gene ALDH7A1 as the probable cause of the seizures, due to a rare metabolic condition. Based on this machine recommendation, doctors were able to swiftly treat the boy with changes to his diet and he was discharged from hospital in under 36 hours, with no apparent ill effects from the seizure episode.
Balancing Innovation with Data Privacy
The path to widespread adoption of AI in healthcare is not, however, straightforward. In a prediction that, in the wake of COVID-19, may prove to be somewhat overly conservative, Lawry [5] warns that the volume of medical and healthcare data is doubling in size every 24 months and will total 2,314 exabytes (EB) in 2020. The forecast rate of data expansion is, however, non-linear and Lawry adds that the amount of global healthcare data will soon be measured in zettabytes (ZB) and yottabytes (YB).
What is clear is that both the volume and complexity of the data being processed in healthcare is increasing dramatically. Topol’s [6] survey of the field foresees the provision of virtual health guidance to patients using AI to resolve appropriate strategies from a combination of the medical corpus, disparate data composed of physical, social, behavioral, genomic, pharmacological, pathological, and biosensor data, and the medical history of the patient and their family. He argues, in respect of patients at risk of developing Type-2 diabetes, that analysis of this multimodal data demonstrates how “smart algorithms that incorporate an individual’s comprehensive data are likely to be far more informative and helpful.”
As evidenced in national responses to the COVID-19 pandemic, private medical data, coupled with contextual data, such as that yielded by contact tracing applications and genomic databases, can provide clinicians and decision-makers with the information necessary to treat and monitor specific diseases. Yet, the wealth of data now available to support the application of AI in healthcare carries with it a series of challenges that must be addressed if the public are to be confident in the benefits provided to patients. Topol [6] counsels that “an overriding issue for the future of AI in medicine rests with how well privacy and security of data can be assured. Given the pervasive problems of hacking and data breaches, there will be little interest in use of algorithms that risk revealing the details of the patient’s medical history.”
The challenge faced by healthcare organizations is reinforced by the 2020 HIMSS Healthcare Cybersecurity Survey [7], which concludes that these organizations “need to make cybersecurity a fiscal, technical, and operational priority” (p.30). HIMSS [8] emphasize that “patient lives depend upon the confidentiality, integrity, and availability of data, as well as reliable and dependable technology infrastructure.”
Pervasive Data Security with Confidential Computing
Robust data security depends on the application of appropriate protection of data throughout its lifecycle. The findings of the HIMSS survey [7] that 11% (N = 19) of respondents reported some form of data breach or leakage and 10% (N = 17) of respondents reported malicious insider activity as the cause of significant security incidents in the preceding twelve are noteworthy. HIMSS observed that the actual levels of these security incidents may be higher than those reported. The survey also reported that intellectual property theft (12% of respondents, N = 14) was also seen as a motivation behind security breaches, observing that “intellectual property theft tends to be underreported because there is a lack of awareness of what constitutes intellectual property and/or a lack of mechanisms for tracking the theft of intellectual property assets” (p. 17). Crucially, the HIMSS survey found that only 73% (N = 123) of respondents had implemented data at rest encryption, with 77% (N = 129) using data in transit encryption to transfer data.
Data encryption is a fundamental security requirement in healthcare. Under the General Data Protection Regulations (GDPR) of the European Union, the penalties for exposure of data where insufficient action has been taken to protect the data can be punitive, with fines, up to a maximum of €20 million or 4% global turnover – whichever is greater. In the USA, under the provisions of the Health Insurance Portability and Accountability Act 1996 (HIPAA), the HIPAA Security Rule defines the security requirements for all individually identifiable health information that is created, received, maintained, or transmitted by a healthcare entity that is subject to the Act. This electronic-protected health information (e-PHI) must be adequately protected against “anticipated impermissible uses or disclosures” [9].
While the importance of protecting data at rest and in transit are (or should be) well understood within the healthcare sector, consideration must also be given to the protection of data in use. Fortanix regards each of these three modes of data protection to be essential components of pervasive data security. Although data encryption at rest using cryptographic keys and secure transmission of data using technologies such as Transport Layer Security (TLS) can prevent an attacker from accessing data from storage or across network communications, the data remains exposed at the point at which it is processed by a designated computer. This vulnerability of data in use is central to the safe implementation of AI solutions in healthcare and has restricted adoption of cloud computing services, where these computing resources are regarded as an untrusted computing platform.
Fortunately, the emergence of Confidential Computing as a new security paradigm offers healthcare data providers, AI application developers, clinicians, and data scientists a solution to the practical problem of protecting e-PHI data when it is being processed. Fortanix Runtime Encryption(R)technology implements Intel(R) Software Guard Extensions (IntelSGX) to provide an automated and auditable solution that will protect complex AI workloads on any enabled platform, whether on-premises or in the cloud.
Confidential Computing [10] uses a hardware-based trusted execution environment (TEE) to provide a protected memory region on the computer processing the AI workload, where data is isolated and protected from potential attackers. The IntelSGX security model creates a memory region referred to as a secure “enclave”, within which sensitive data and application code is protected from the host operating system, hypervisor, malicious root user, and peer applications running on the same processor. All data being processed by an application deployed within the enclave is encrypted prior to being passed to dynamic memory and attestation services can be used to validate the provenance of data and the integrity of the application code within the enclave [11].
Protecting Healthcare AI Workloads with Confidential Computing
The Confidential Computing technology developed by Fortanix, combined with the data at rest protection offered by our Self-Defending Key Management Service TM (KMS), provides healthcare organizations with the ability to protect both e-PHI data and the intellectual property contained in AI algorithms, even on untrusted infrastructure.
Using the attestation properties of Intel SGX, data providers can verify the integrity of the consuming AI application, before sending their data via a TLS pathway to the secure enclave – with the TLS connection terminating inside of the enclave, to prevent any exposure of the data outside of this environment. Since the input data should be encrypted at rest, it is necessary for the AI application to decrypt the data prior to processing. Fortanix Confidential Computing Manager automates enclave attestation and provides the application with a certificate that can subsequently be used to authenticate to the Fortanix Self-Defending KMS. Once authentication is completed, the data encryption key can be used by the AI application to decrypt the sensitive healthcare data for use by the model characterizing the workload. Throughout the runtime of the algorithm, data is always encrypted in memory and only ever processed unencrypted within the enclave. Hence, any attempt to implement a memory scraping attack results in retrieval of encrypted data that retains the protection of the healthcare data required by legal frameworks such as HIPAA and GDPR. An attempt to access the enclave to retrieve data during processing is prevented by the isolation provided by the TEE. Implementing encryption of the results of the AI algorithm, within the boundary of the enclave, before the secure transfer of the output data to disk or to a downstream application, ensures complete end-to-end protection of the healthcare data being processed and the intellectual property within the application code deployed to the enclave. A model workflow employing Fortanix products to provide security for healthcare AI with Confidential Computing is represented in Figure 1:
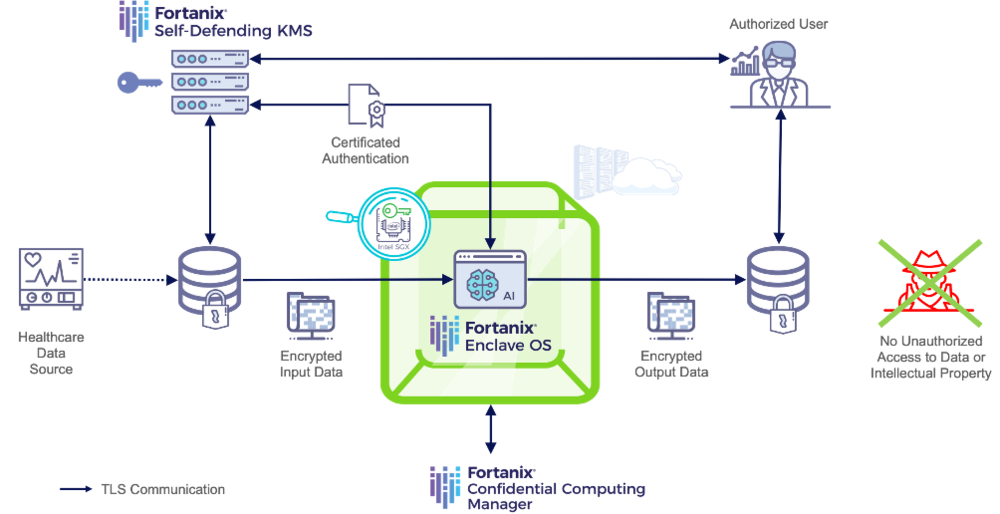
Figure 1: Protection of healthcare AI workflows using Fortanix Confidential Computing technology.
To illustrate such a solution in practice, we have produced a short demonstration that features secure deployment of the DarkCovidNet CNN reported by Ozturk et al. [12] within an Intel SGX enclave, with data at rest encryption of both the sample chest X-ray data [13][14] used to train the neural network and the results of the training cycle. Contrasting the secure implementation of the DarkCovidNet code [15] with unprotected execution of this neural network, we demonstrate how sensitive data remains vulnerable in memory without the protection afforded by Confidential Computing.
A video recording of our implementation of the DarkCovidNet CNN using Confidential Computing can be viewed at: https://www.fortanix.com/blog/2020/12/securing-healthcare-ai-with-confidential-computing/
Conclusions
Accenture has described AI as “healthcare’s new nervous system” [16] with the AI health market expected to experience compound annual growth of 40% per annum. Of their principal findings, Accenture reported that every electronic health record breached is likely to incur a cost of $355. Importantly, breach of private healthcare data has a significant negative impact on consumer trust, undermining the potential benefits to be realized through adoption of AI technologies.
Using Confidential Computing technology, Fortanix can provide global healthcare providers with the seamless and scalable data security that is required by the complex workloads associated with AI in clinical settings. By integrating auditable security throughout the AI workflow, as demonstrated for the DarkCovidNet neural network using the combined application of Confidential Computing and data at rest encryption, healthcare organizations can now benefit from the data security necessary to reduce the development time of new AI solutions, improve the clinical out-comes for patients, realize new medical breakthroughs, and establish a platform for the continued response to COVID-19 and other, as yet unforeseen, public health requirements.
List of References
[1] DeepMind, 2020. AlphaFold: a solution to a 50-year-old grand challenge in biology. Available at: https://deepmind.com/blog/article/alphafold-a-solution-to-a-50-year-old-grand-challenge-in-biology [Accessed: 6 December 2020].
[2] Wrapp, D., Wang, N., Corbett, K. S., Goldsmith, J. A., Hsieh, C.-L., Abiona, O., Graham, B. S., McLellan, J. S., 2020. Cryo-EM of the 2019-nCov spike in the prefusion conformation. Science, 365(6483), pp. 1260-1263.
[3] DeepMind, 2020. Computational predictions of protein structures associated with COVID-19. Available at: https://deepmind.com/research/open-source/computational-predictions-of-protein-structures-associated-with-COVID-19 [Accessed: 6 December 2020].
[4] Topol, E., 2019. Deep medicine: How artificial intelligence can make healthcare human again. New York: Basic Books.
[5] Lawry, T., 2020. Artificial intelligence in healthcare: a leader’s guide to winning in the new age of intelligent health systems. Boca Raton: CRC Press.
[6] Topol, E., 2019. High-performance medicine: the convergence of human and artificial intelligence. Nature Medicine, 25, pp. 44-56.
[7] Healthcare Information and Management Systems Society (HIMSS), 2020. 2020 HIMSS Cybersecurity Sur-vey. Available at: https://www.himss.org/sites/hde/files/media/file/2020/11/16/2020_himss_cybersecurity_survey_final.pdf [Accessed: 6 December 2020].
[8] https://www.himss.org/resources/himss-healthcare-cybersecurity-survey [Accessed: 6 December 2020].
[9] https://www.cdc.gov/phlp/publications/topic/hipaa.html [Accessed: 6 December 2020].
[10] Confidential Computing Consortium, 2020. Confidential Computing Deep Dive v1.0. The Linux Foundation. Available at: https://confidentialcomputing.io/wp-content/uploads/sites/85/2020/10/Confidential-Computing-Deep-Dive-white-paper.pdf [Accessed: 6 December 2020].
[11] Johnson, S., Scarlata, V., Rozas, C., Brickell, E., McKeen, F., 2016. Intel Software Guard Extensions: EPID provisioning and attestation services. Available at: https://software.intel.com/sites/default/files/managed/57/0e/ww10-2016-sgx-provisioning-and-attestation-final.pdf [Accessed: 6 December 2020].
[12] Ozturk, T., Talo, M., Yildirim, E. A., Baloglu, U. B., Yildirim, O., Acharya, U. R., 2020. Automated detection of COVID-19 cases using deep neural networks with X-ray images. Computers in Biology and Medicine, 121, 103792.
[13] https://github.com/ieee8023/COVID-chestxray-dataset [Accessed: 6 December 2020].
[14] https://www.kaggle.com/paultimothymooney/chest-xray-pneumonia [Accessed: 6 December 2020].
[15] https://github.com/muhammedtalo/COVID-19/blob/master/DarkCovidNet%20%20for%20binary%20classes.ipynb [Accessed: 6 December 2020].
[16] Accenture, 2020. Artificial intelligence: healthcare’s new nervous system. Available at: https://www.accenture.com/_acnmedia/PDF-49/Accenture-Health-Artificial-Intelligence.pdf#zoom=50 [Accessed: 6 December 2020].
0 comments
Leave a comment
Please log in or register to post a comment